Enter the Era of Accuracy
Sales forecasting is undergoing a significant change, thanks to the integration of artificial intelligence (AI) and big data analytics. Traditional methods, which relied heavily on historical sales data and intuition, often yielded accuracy rates between 60% and 79%. These conventional techniques are increasingly being outpaced by the capabilities of AI-driven models. AI in sales forecasting uses large amounts of data to improve accuracy, achieving rates as high as 95%.
The Power of AI in Sales Forecasting
With the help of AI and big data, organizations can analyze complex datasets from various sources such as CRM systems, social media platforms, and even weather data. This combination not only enhances accuracy but also provides real-time insights that are essential for quick decision-making.
One example of this is Prophet, a tool created by Facebook that uses advanced algorithms to predict future sales trends with impressive precision. Additionally, the rise of mobile technology allows sales teams to access these predictive insights at any time and from anywhere, further improving their decision-making abilities.
Why Sales Leaders Should Embrace AI
Sales leaders need to understand that using AI and big data is no longer a choice, but a must if they want to succeed in today’s world. This strategy allows for better decision-making using data-driven insights, which gives businesses an edge in an ever-changing market.
As companies transition into this new way of doing things, AI becomes essential in improving key performance indicators (KPIs) and fine-tuning sales strategies for future growth.
Understanding the Bigger Picture
The shift towards AI-powered sales forecasting is just one aspect of a larger trend in technology adoption. A significant event in tech history was the Mother of All Demos, which showcased the potential of computer technology in ways that were once considered impossible. As we embrace these new innovations, it’s crucial to understand their impact on our industries.
The Evolution of Sales Forecasting
Sales forecasting has changed significantly over the years, evolving from basic methods to advanced predictive analytics. Traditional forecasting methods relied heavily on historical sales data, market trends, and a degree of personal intuition. Typically, these approaches achieved accuracy rates between 60% and 79%, often falling short in dynamic market environments.
Traditional Methods: The Foundation of Sales Forecasting
Historical methods such as moving averages, exponential smoothing, and basic trend analysis were the cornerstone of sales forecasting. While these techniques provided a foundation, they were limited by their linear nature and inability to account for non-linear relationships or external variables influencing sales outcomes.
Predictive Analytics: A Game Changer in Forecasting
Predictive analytics has become a game changer in improving the accuracy of forecasts. Unlike traditional methods, which have their limitations, predictive models use large amounts of data and advanced statistical techniques to make more precise predictions. These models go beyond simply recognizing patterns in historical data; they also use that information to forecast future events with greater accuracy.
Key Components of Predictive Analytics in Sales Forecasting
- Predictive Models: These models use historical data and algorithms to find complex patterns that humans might not be able to see.
- Algorithmic Advancements: New algorithms can analyze both straight-line (linear) and curved (non-linear) relationships within data sets, providing insights that older methods might overlook.
- Data Integration: Predictive analytics allows for the combination of different types of data from various sources such as CRM systems, social media, and external economic indicators. This integration helps improve the accuracy of predictions.
The Impact of Predictive Analytics on Sales Forecasting
The integration of predictive analytics into sales forecasting has effectively transformed how businesses anticipate future performance. The reliance on statistical models allows organizations to make data-driven decisions with confidence, paving the way for enhanced strategic planning and resource allocation.
This evolution signals a pivotal shift in business practices where accuracy is no longer a luxury but a necessity. As businesses continue to navigate an increasingly competitive landscape, embracing advanced analytical tools becomes essential in maintaining relevance and achieving sustainable growth.
Beyond Technology: The Leadership Approach
This shift in business practices is not just about adopting new technologies; it also involves a leadership approach that encourages innovation and adaptability.
Understanding Model Collapse
Furthermore, understanding model collapse in predictive analytics is crucial for businesses to avoid potential pitfalls in their forecasting processes.
The Role of AI in Sales Forecasting Accuracy
AI-driven forecasting has transformed the landscape of sales predictions by significantly enhancing accuracy. Traditional methods often fell short, achieving only 60-79% accuracy. In contrast, some AI models now reach up to 95% accuracy, setting a new benchmark in predictive reliability.
How AI Improves Sales Forecasting
AI uses advanced machine learning algorithms that can find intricate patterns and connections in large datasets. These algorithms can pick up on subtle trends and relationships that a human analyst might miss. By analyzing extensive amounts of data from various sources like customer interactions, market trends, and transaction records, AI provides a deeper understanding of the sales landscape.
Continuous Learning for Adaptability
One of the unique advantages of AI is its ability to continuously learn from new data inputs. When new information comes in, AI models improve their predictions by adjusting to new trends and shifts in the market. This ongoing learning process keeps predictions up-to-date and precise, giving businesses an advantage over their competitors.
Using AI for Better Decision-Making
AI in sales forecasting not only makes predictions more accurate but also provides valuable insights. With these improved abilities to predict outcomes, organizations can make confident decisions, resulting in better business results. Instead of relying only on past data, businesses are now adopting a flexible approach where real-time analysis influences their future plans.
Empowering Data-Driven Decision-Making
AI enables businesses to use data-driven insights for better decision-making. With the help of advanced algorithms and continuous learning, companies can effectively and quickly navigate the complexities of today’s markets. This move towards AI also highlights the significance of leadership in successfully adopting these technologies.
Building the Right Skills for Implementation
Moreover, it’s essential for organizations to find the right skills within their teams to fully leverage the potential of AI in sales forecasting. This involves not just technical skills related to data analysis and machine learning but also soft skills such as adaptability and strategic thinking which are crucial in this rapidly evolving landscape.
Benefits of Implementing AI-Driven Sales Forecasting Solutions
The integration of AI into sales forecasting offers numerous advantages that dramatically enhance the precision and effectiveness of sales strategies.
Improved Accuracy
AI algorithms can analyze vast amounts of data and identify patterns that may not be apparent to human analysts. This leads to more accurate sales predictions and better decision-making.
Increased Efficiency
By automating routine tasks, organizations can significantly boost efficiency within their sales teams. This automation minimizes the need for manual data processing, thereby reducing the likelihood of errors and freeing up valuable time for more strategic endeavors.
Enhanced Flexibility
AI-driven solutions can adapt to changing market conditions and customer preferences. This flexibility allows businesses to stay ahead of the competition and respond quickly to new opportunities.
Better Resource Allocation
With accurate sales forecasts, organizations can allocate their resources more effectively. This includes optimizing inventory levels, managing production schedules, and planning marketing campaigns.
Improved Collaboration
AI-powered tools can facilitate collaboration between different departments involved in the sales process. This ensures that everyone is working towards a common goal and increases overall productivity.
1. Efficiency Improvements Through Automation
AI’s ability to handle large amounts of data quickly enables sales teams to automate repetitive tasks like data entry and report generation. This automation not only speeds up the workflow but also minimizes human errors, leading to more accurate data processing. As a result, sales personnel can concentrate on essential activities that require human insight and creativity, such as developing strategies and managing client relationships.
2. Bias Reduction in Predictions
Traditional sales forecasting methods often suffer from biases introduced by human subjectivity. AI-driven solutions provide an objective approach by analyzing data trends without preconceived notions. Machine learning models leverage historical data to identify patterns free from human influence, ensuring that forecasts are based on empirical evidence. This objectivity leads to more accurate predictions, enabling businesses to make informed decisions with confidence.
Additionally, these AI-driven forecasting solutions represent a significant leap forward in the realm of sales strategy execution. By harnessing the power of big data and machine learning, companies can anticipate market dynamics with unprecedented accuracy. As a result, they become better equipped to respond swiftly to changing conditions and maintain a competitive edge in an increasingly data-driven landscape.
For those interested in exploring more about the methodologies behind these AI solutions, resources like this could provide valuable insights. Also, if you’re seeking motivation during this transformative journey, consider reflecting on some motivational quotes that might inspire you.
Real-Time Insights for Agile Decision-Making with AI
The ability of AI systems to process information in real-time has revolutionized sales management. Now, teams can receive instant insights and make swift decisions right away. With these advanced technologies, sales teams can monitor their pipeline effectively and adapt promptly to shifts in the market and customer requirements.
1. Instant Updates
AI-driven platforms offer continuous monitoring and analysis of sales pipelines. This real-time scrutiny ensures that any changes or trends are immediately identified, enabling teams to adjust strategies proactively rather than reactively. The effectiveness of these platforms often relies on robust API architecture, which allows seamless integration and data exchange between different software applications.
2. Agile Decision-Making
With real-time data available, sales managers can make informed decisions that align with current market conditions. This flexibility is crucial in today’s fast-paced business environment where delays can lead to missed opportunities. The ability to use technical expertise within the team further improves this decision-making process, allowing for a better understanding of the data and its effects.
3. Enhanced Responsiveness
The ability to access real-time data allows organizations to quickly adapt to changes in consumer behavior or competitor actions, staying ahead in fast-paced markets. This level of responsiveness is crucial, especially when dealing with unpredictable market situations often shown in made-up stories used for planning and predicting.
These abilities are a major improvement over old-fashioned ways, enabling organizations to use data effectively for making strategic choices. By incorporating real-time information into their processes, companies not only become more responsive but also boost their overall performance and customer satisfaction.
Using Big Data Analytics to Improve Sales Strategies
The rise of big data analytics tools and technologies has changed the way businesses approach sales. These tools provide valuable insights into consumer behavior and market trends, allowing organizations to make informed decisions on a larger scale.
Here are some ways big data analytics can enhance sales strategies:
1. Data Integration
Businesses can gather information from various sources such as CRM systems, social media platforms, and e-commerce websites. By combining this data, companies can get a complete picture of their market, helping them identify trends and patterns that were previously hidden.
2. Advanced Analytical Capabilities
Big data analytics uses complex algorithms that can analyze both simple and complex relationships within datasets. This allows sales teams to extract useful insights and create strategies that are more in line with current market conditions.
3. Scalability and Efficiency
Big data analytics can handle large amounts of data, ensuring that organizations can operate efficiently even as they grow. This ability to scale is crucial in today’s fast-paced markets where quick decision-making is essential for success.
4. Predictive Insights
In addition to analyzing past data, big data also uses predictive models to forecast future trends. By understanding potential changes in customer preferences or competitor actions, companies can proactively adjust their sales strategies.
By using big data analytics strategically, organizations gain a competitive advantage by not only meeting current demands but also anticipating future opportunities and challenges. This proactive approach improves their ability to create strong sales strategies that align with changing market conditions.
Customer Segmentation and Targeting with Big Data Insights
Customer segmentation is a critical component of sales strategies, especially in today’s competitive marketplace. It involves dividing a broad target audience into smaller, more defined groups based on specific characteristics or behaviors. This approach allows businesses to tailor their marketing efforts and messages to resonate with each segment, increasing the chances of conversion and customer loyalty.
Understanding and reaching the right audience is crucial for success. Big data analytics plays a pivotal role in this process by enabling businesses to identify distinct customer segments with precision.
1. Data-Driven Segmentation
By analyzing large amounts of data from various sources like CRM systems and social media platforms, companies can identify patterns and trends that define different customer groups. This segmentation enables the creation of customized marketing strategies that appeal to specific audiences.
2. Improved Sales Forecasting
Using big data, organizations can predict more accurately which customer groups are likely to be interested in their products or services. This ability to foresee helps in refining marketing strategies and using resources efficiently.
3. AI and Future Use of Big Data
As AI continues to evolve, its integration with big data analytics will further refine customer targeting efforts. Machine learning algorithms can uncover hidden correlations within datasets, offering deeper insights into consumer behavior and preferences.
Big data analytics not only enhances the precision of customer segmentation but also empowers businesses to implement more targeted and effective sales strategies. This capability ensures that marketing efforts are aligned with the evolving needs and expectations of diverse consumer bases.
Optimizing Resource Allocation through Predictive Analytics
Sales organizations often face challenges in allocating resources effectively, which is crucial for meeting sales targets. Predictive analytics proves to be an invaluable tool in this situation, providing a data-driven approach that improves decision-making abilities.
Understanding Predictive Analytics
Predictive analytics uses past data and advanced algorithms to predict future trends and outcomes. This technique empowers sales teams to:
- Identify High-Potential Leads: With the help of predictive models, organizations can analyze large datasets to find leads with the highest chances of conversion. This enables sales teams to focus their efforts on prospects who are most likely to result in successful transactions, making better use of their time and resources.
- Target Lucrative Markets: In addition to individual leads, predictive analytics can also examine wider market trends to identify regions or sectors with significant growth potential. By understanding these dynamics, companies can allocate their resources more strategically, concentrating on areas that promise higher returns.
- Evaluate Resource Needs: The insights gained from predictive analysis provide a clearer understanding of resource requirements across different segments. This helps in aligning sales personnel, marketing budgets, and operational support where they are most necessary, minimizing waste and improving efficiency.
The Impact of Predictive Analytics on Resource Allocation
Sales organizations can transform their operations by integrating predictive analytics into their resource allocation processes. Instead of relying solely on reactive strategies, businesses can adopt foresight-driven planning by leveraging predictive analytics.
This shift not only optimizes existing resources but also positions companies for long-term growth in competitive markets. As the business landscape continues to evolve, being able to anticipate needs and take appropriate action will remain a key factor that sets apart forward-thinking enterprises from others.
Considering External Factors for More Accurate Sales Forecasts
Sales performance is not solely determined by internal metrics and historical data. External factors influencing sales performance are crucial in shaping the accuracy of forecasts. Incorporating these elements allows businesses to construct more robust predictive models.
1. Economic Trends
Economic indicators like GDP growth rates, unemployment levels, and consumer confidence indices give a broad view of the economy that can greatly impact sales results. For example, when the economy is struggling, people usually spend less, which in turn affects sales numbers in different industries.
2. Competitive Landscape
Understanding what competitors are doing and how they are positioning themselves is crucial. Changes in market share, pricing strategies, and new product launches by competitors can influence consumer preferences and demand patterns. By considering these competitive actions, organizations can modify their forecasts to better predict changes in the market.
3. Regulatory Changes
Changes in regulations can affect operational costs and market access. Industries like pharmaceuticals or financial services often see fluctuations due to shifts in compliance requirements or trade policies.
These external factors need to be combined with traditional forecasting methods to improve prediction accuracy. Using big data analytics allows organizations to include these variables in real-time, thus improving their forecasting abilities and getting ready for unforeseen market changes. This all-encompassing strategy ensures that sales plans stay flexible and strong in the face of changing external factors.
Making Actionable Recommendations with Prescriptive Analytics
Prescriptive analytics techniques applied to sales data are changing the way sales strategies are developed. While traditional forecasting methods mainly focus on predicting future outcomes, prescriptive analytics goes a step further by providing specific recommendations based on past trends and data analysis.
Key Benefits of Prescriptive Analytics in Sales
- Informed Decision-Making: By leveraging AI and advanced computational models, prescriptive analytics can analyze vast datasets to identify optimal strategies. This approach allows businesses to not only predict outcomes but also understand the necessary steps to achieve desired results.
- Scenario Analysis: Businesses can simulate various scenarios based on historical data, evaluating the potential impact of different strategies. This capability aids in selecting the most effective course of action under varied market conditions.
- Resource Optimization: Prescriptive analytics provides insights into efficient resource allocation. By identifying high-potential leads or markets, companies can direct their efforts and resources where they are likely to yield the highest returns.
Consider a company utilizing prescriptive analytics for its sales operations. The system might analyze past interactions, purchase histories, and external factors such as economic trends to recommend specific marketing campaigns or target customer segments that align with business objectives.
Future Use of Big Data and AI
As the industry continues to change, combining big data with prescriptive analytics will be essential for staying competitive. The ability to turn complex data into strategic actions allows organizations to quickly adjust to shifting market conditions, ensuring ongoing growth and flexibility in decision-making processes.
Future Sales Methods Powered by Generative AI and Automation
Generative AI is changing industries by offering new solutions that improve efficiency, creativity, and decision-making. In fields such as banking and technology, generative AI applications are transforming operations through automated content creation, personalized customer interactions, and predictive analytics. These advancements signal significant changes in future sales methods, allowing organizations to leverage the power of AI to redefine their sales strategies.
Generative AI Applications Across Industries
1. Banking
Generative AI is used in the banking sector for various purposes such as:
- Fraud detection
- Risk assessment
- Personalized financial advice
By analyzing large amounts of data, these systems can:
- Identify unusual patterns that indicate fraudulent activity
- Provide tailored investment recommendations based on individual client profiles
2. Technology
In the technology industry, companies utilize generative AI for:
- Automating software development processes
- Improving virtual assistants
- Strengthening cybersecurity measures
These applications help streamline complex tasks, minimize human errors, and enhance user experiences by offering highly customized solutions.
Impact on Future Sales Methods
The integration of generative AI into sales operations paves the way for transformative changes in how businesses approach their sales strategies. By automating routine tasks and optimizing resource allocation, companies can focus more on strategic initiatives that drive growth.
How Automation Can Boost Sales Team Productivity
1. Lead Scoring
Automation tools powered by machine learning algorithms can analyze potential leads’ behavior patterns to assign scores indicating their likelihood to convert. This process enables sales teams to prioritize high-potential prospects effectively.
2. Proposal Generation
Automated systems can generate customized proposals based on client data and preferences. This capability not only saves time but also ensures consistency and accuracy in proposal content.
AI-driven automation empowers sales teams by reducing the burden of mundane tasks, allowing them to concentrate on building relationships with clients and crafting strategies that align with organizational goals. As generative AI continues to evolve, its applications will undoubtedly redefine traditional sales methods, ushering in a new era characterized by enhanced productivity and innovation.
In this AI tool-driven environment, the role of sales leadership is also evolving. Leaders must now adapt to these technological advancements while still maintaining a human touch in their interactions with clients.
How AI Tools Are Changing Sales Roles
In today’s fast-paced sales world, AI tools are essential in redefining traditional roles. These tools automate repetitive tasks, allowing salespeople to focus on strategic activities that directly impact business results.
What Tasks Can AI Tools Handle?
- Data entry
- Scheduling
- Lead qualification
By handling these tasks efficiently, AI systems enable sales teams to concentrate on building relationships and creating personalized strategies for clients.
The Benefits of AI-Enabled Platforms for Managers
AI-enabled platforms offer real-time feedback and coaching capabilities that are invaluable for managers aiming to enhance team performance. These platforms analyze large amounts of data to evaluate individual and team performance, providing insights that inform specific coaching actions. Managers receive practical feedback on areas needing improvement, allowing them to implement customized coaching strategies on a larger scale. This data-driven approach ensures that sales teams continually refine their methods and attain greater levels of productivity.
How AI Tools Foster Strategic Thinking
The integration of AI tools into sales roles not only streamlines operations but also fosters an environment where strategic thinking is prioritized. Sales professionals benefit from the ability to concentrate on activities that require human creativity and judgment, such as negotiating deals and understanding nuanced customer needs. Meanwhile, managers leverage AI-generated insights to refine coaching techniques, promoting a culture of continuous improvement within the organization.
The shift towards AI-enhanced roles represents a fundamental change in how sales teams operate, emphasizing efficiency and strategic engagement over manual processes. As organizations continue to adopt these innovative solutions, the potential for driving sustained growth and competitive advantage becomes increasingly evident.
Challenges Organizations Face When Implementing AI for Sales Forecasting
Integrating AI into sales forecasting comes with its own set of challenges. Organizations looking to leverage AI and big data need to overcome several common hurdles during the adoption process.
Data Quality Issues
To ensure the success of AI-driven sales forecasting, it is crucial to address data quality first. The effectiveness of AI models heavily relies on the cleanliness and completeness of the data fed into them. Incomplete or inaccurate data can lead to flawed predictions, diminishing the potential benefits of AI applications in sales forecasting.
Companies must establish strict data management protocols to maintain high standards of data quality. This includes:
- Regular audits and cleansing processes to remove duplicate or erroneous entries.
- Implementation of robust data governance frameworks to ensure consistency and accuracy.
- Investment in technologies that facilitate seamless data integration from various sources.
Resistance to Change
Another significant hurdle is the resistance from employees who may be skeptical about relying on machines for critical decision-making processes. The introduction of AI can lead to apprehension among staff members accustomed to traditional methods, fearing job displacement or a loss of control over decision-making.
To alleviate these concerns, organizations can:
- Provide comprehensive training programs that emphasize the complementary role of AI, highlighting how it enhances rather than replaces human expertise.
- Foster an organizational culture that embraces innovation and change by showcasing successful case studies where AI has improved outcomes.
- Ensure transparent communication about the goals and expected benefits of AI adoption, involving employees in the transition process.
By addressing these challenges head-on, organizations can better position themselves to capitalize on the transformative potential of AI in sales forecasting, paving the way for enhanced accuracy and efficiency in their operations.
Balancing Human Expertise with Machine Predictions: A Key Consideration
In the ever-changing world of sales forecasting, it’s crucial to find a balance between human expertise and machine predictions. While AI tools offer unmatched accuracy, they can’t fully replace the deep understanding that experienced professionals bring. That’s where hybrid forecasting approaches come in, combining the best of both worlds.
How Human Expertise Adds Value
Human experts contribute to the forecasting process in several ways:
- Contextual Insights: Professionals interpret forecasts within the larger context of market dynamics and company strategies.
- Intuition and Experience: Seasoned sales experts can often predict trends based on their years of industry experience, something AI models may not fully replicate.
- Ethical Considerations: Humans can assess the ethical implications of decisions suggested by AI, ensuring alignment with organizational values.
The Advantages of Machine Predictions
On the other hand, machine predictions have their own set of benefits:
- Data-Driven Accuracy: Algorithms analyze large datasets to find patterns and trends that are invisible to humans.
- Efficiency in Repetitive Tasks: Automation reduces manual work, allowing professionals to focus on strategic initiatives.
- Continuous Learning: AI models adapt quickly to changes by learning from new data inputs.
An effective hybrid approach combines these strengths, resulting in more reliable sales forecasts. This integration not only improves decision-making but also helps organizations stay flexible and competitive in a constantly changing market.
Strategies for Managing Change Within Organizations During AI Implementation Phases
Implementing AI solutions for sales forecasting requires a comprehensive change management strategy. Organizations must address potential resistance and ensure seamless integration of new technologies to maximize benefits.
Key Change Management Strategies:
- Communication and Awareness: Regular communication is essential to keep all stakeholders informed about the AI implementation process. This involves explaining the benefits, expected outcomes, and how it will enhance current sales processes. Clear messaging helps align organizational goals with individual roles.
- Training and Education: Providing adequate training sessions tailored to different levels of expertise ensures that employees understand how to utilize AI tools effectively. Training should focus on both technical skills and the strategic value AI brings to sales forecasting.
- Leadership Involvement: Active involvement from leadership can foster a culture of innovation and trust. Leaders should champion the AI initiatives, setting an example by embracing the technology themselves and encouraging others to do the same.
- Feedback Mechanisms: Establishing channels for feedback allows employees to voice concerns and suggestions regarding the AI integration process. This not only aids in fine-tuning the implementation but also promotes a sense of ownership among team members.
- Incremental Rollout: Adopting a phased approach rather than a full-scale rollout can mitigate risks associated with technological disruptions. It allows time for adjustments based on initial feedback, ensuring a smoother transition.
- Incentive Programs: Offering incentives or rewards for early adopters can motivate employees to engage with the new technology enthusiastically. Recognizing efforts encourages widespread acceptance across teams.
Adopting these strategies enhances adaptability, ensuring that organizations stay competitive in an era where AI-driven forecasting is crucial for success.
Training Employees to Understand Data: A Crucial Step for Successful AI Adoption
The use of AI-powered sales forecasting and predictive analytics is changing the way companies work. However, for these advanced technologies to be successfully integrated, it is essential to train employees on how to understand complex analytical results. As the sales industry evolves, training programs that improve employees’ ability to work with intricate data become increasingly important.
1. Understanding Advanced Modeling Techniques
Employees need to be skilled at understanding and interpreting outputs from complex modeling techniques. Predictive analytics in sales forecasting uses statistical algorithms and historical data to generate forecasts with improved accuracy. Training programs should focus on explaining these methods, enabling personnel to decipher patterns and relationships identified by AI.
2. Bridging the Skills Gap
The shift towards AI and big data needs a workforce skilled in these fields. Companies should invest in extensive training programs that include key topics like data interpretation, statistical analysis, and insights extraction. This will help close the skills gap and foster an environment where employees are comfortable using AI tools to make informed decisions.
3. Enhancing Critical Thinking and Analytical Skills
In addition to technical knowledge, training should also emphasize critical thinking and analytical skills. Employees need to be able to effectively assess the implications of data-driven insights and extract actionable information from complex datasets. Developing these abilities will empower individuals to contribute strategically in their roles.
4. Tailored Training Approaches
Customized training approaches are important for ensuring relevance and effectiveness. Different roles within an organization may require varying levels of proficiency in working with sales forecasts and big data analytics. Tailored programs can address specific needs and align educational content with departmental objectives.
Investing in employee development through targeted training initiatives not only improves individual skills but also strengthens organizational capabilities. As companies adopt AI and big data, it is crucial to cultivate a workforce that can understand these technologies in order to maintain a competitive edge in an increasingly data-driven world.
Transforming KPI Tracking with Real-Time Visibility Powered by Advanced Technologies
In the fast-paced world of sales, being able to track Key Performance Indicators (KPIs) in real-time has become crucial for success. Modern technology solutions specifically designed for this purpose offer numerous benefits, fundamentally changing how sales teams monitor and react to their performance metrics.
The Importance of Real-Time Visibility
Real-time visibility is essential for sales teams to respond quickly to challenges and opportunities. By providing immediate access to KPIs such as conversion rates, lead times, and sales volumes, these advanced technologies empower decision-makers with up-to-the-minute insights. This immediacy ensures that teams can adapt strategies swiftly, aligning their efforts with dynamic market demands.
The Benefits of Real-Time KPI Tracking
Key advantages of real-time KPI tracking include:
- Enhanced Decision-Making: Sales leaders equipped with current data can make informed decisions quickly, leading to better resource allocation and prioritization.
- Proactive Issue Resolution: Identifying potential problems before they escalate allows teams to implement corrective actions rapidly, minimizing adverse impacts on performance.
- Performance Optimization: Continuous monitoring enables ongoing assessment of strategies and tactics, fostering a culture of perpetual improvement within the organization.
How Advanced Technologies Enable Real-Time Tracking
The use of advanced tools like AI-powered dashboards and predictive analytics platforms makes this level of visibility possible. These systems gather data from various sources and analyze it instantly to provide actionable insights. As a result, sales teams can quickly adapt and stay competitive by using up-to-date information for strategic decision-making.
The Future of KPI Tracking in Sales
As digital transformation continues, organizations must embrace technologies that offer real-time KPI tracking to stay competitive in a data-driven world. The focus on immediate access to performance metrics highlights the importance of integrating these technologies into business operations.
Improved Team Collaboration with Advanced Technology for Better KPI Tracking
Advanced technologies have greatly improved the way organizations track their performance, leading to better collaboration between different teams. This collaboration is essential for optimizing the entire value chain process, which includes various areas such as manufacturing, logistics, distribution, and marketing.
By using modern technology solutions designed to capture important metrics, organizations can ensure smooth cooperation between departments. This, in turn, leads to increased profitability and sustainable growth.
Key Benefits of Cross-Functional Alignment
- Improved Communication and Collaboration: Enhanced tracking capabilities facilitate real-time data sharing among departments. This transparency ensures that all functional areas work cohesively towards common objectives, reducing misunderstandings and fostering a culture of collaboration.
- Streamlined Processes: With precise metrics available at their fingertips, teams can identify inefficiencies and bottlenecks more effectively. This insight allows for timely interventions and process optimization, which is particularly valuable in dynamic sectors like manufacturing and logistics.
- Enhanced Decision-Making: Real-time visibility into key performance indicators (KPIs) enables managers to make informed decisions quickly. By aligning strategies with accurate data, organizations can respond proactively to market changes, enhancing their competitive edge.
- Unified Strategic Goals: When every department has access to consistent and relevant data, aligning departmental goals with the organization’s overarching objectives becomes more straightforward. This unity is essential for achieving long-term sustainability and growth.
Role of AI and Big Data in Forecasting
AI and Big Data play a crucial role in improving sales forecasting and driving collaboration between different departments. Here’s how they make an impact:
1. Sales Forecasting
AI models provide insights that inform sales strategies by predicting customer behavior patterns and potential market shifts.
2. Supply Chain Optimization
Big data analytics improve inventory management by predicting demand fluctuations and streamlining supply chain operations.
3. Marketing Strategies
Tailored marketing initiatives benefit from big data insights by targeting specific customer segments more effectively.
Future Prospects
As organizations continue to use AI and big data, the future looks promising with even more collaboration between different departments. Being able to accurately predict results in various business areas will give companies the power to constantly innovate while staying true to their strategic objectives.
This new age of accuracy not only improves how things work but also makes sure that every department plays its part in achieving long-term success. This is crucial in today’s business world.
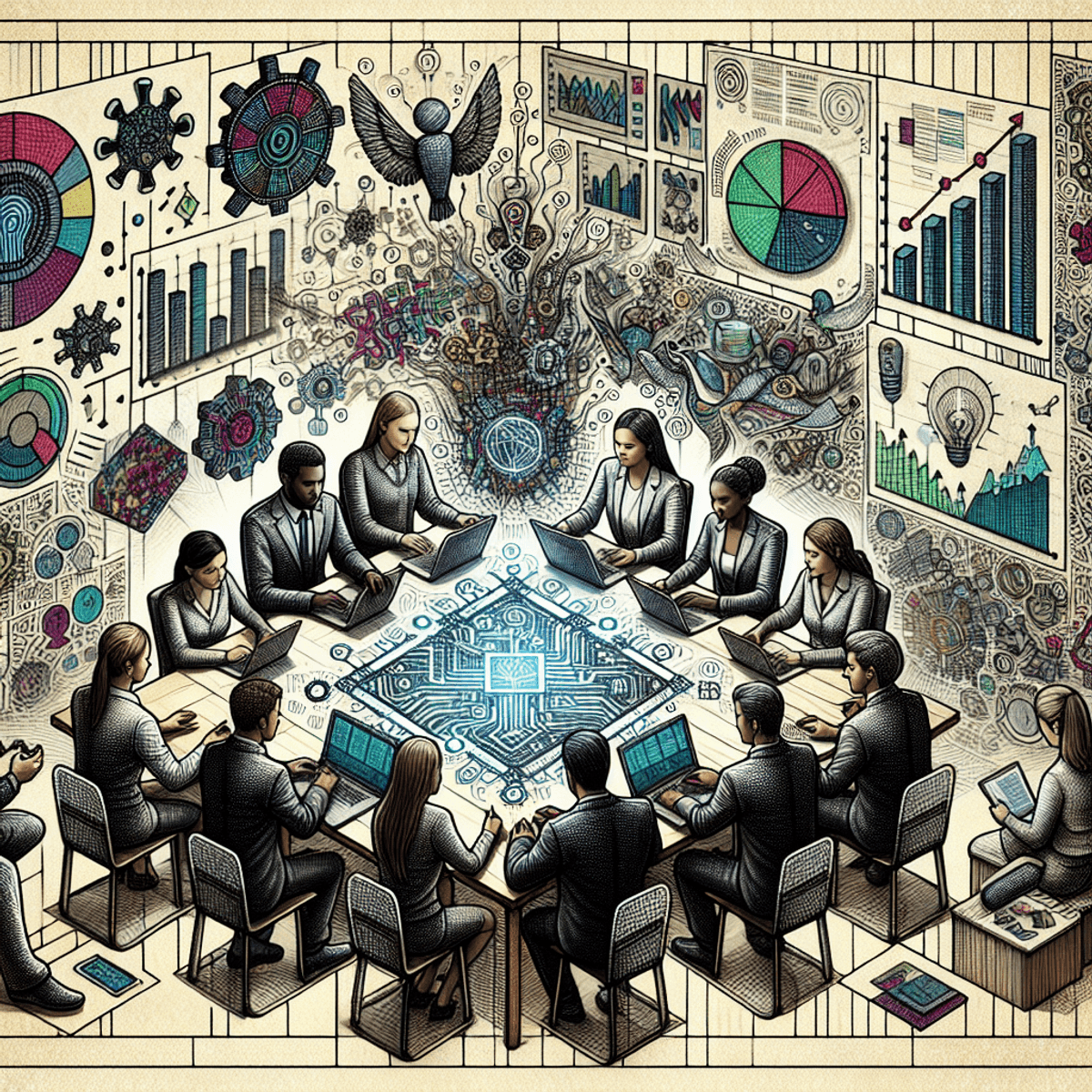
Explore Model Collapse in AI: its causes, impacts on performance, and the role of synthetic data in ensuring reliability.